队列
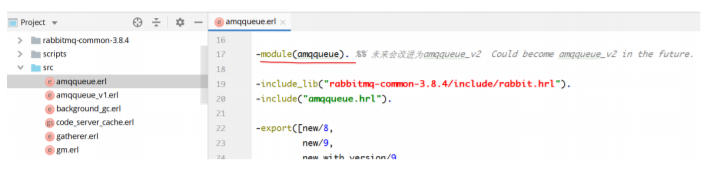
声明队列记录:
-record(amqqueue, {
name :: rabbit_amqqueue:name() | '_', %% immutable
durable :: boolean() | '_', %% immutable
auto_delete :: boolean() | '_', %% immutable
exclusive_owner = none :: pid() | none | '_', %% immutable
arguments = [] :: rabbit_framing:amqp_table() | '_', %% immutable
pid :: pid() | ra_server_id() | none | '_', %% durable (just so we
%% know home node)
slave_pids = [] :: [pid()] | none | '_', %% transient
sync_slave_pids = [] :: [pid()] | none| '_',%% transient
recoverable_slaves = [] :: [atom()] | none | '_', %% durable
policy :: binary() | none | undefined | '_', %% durable, implicit
%% update as above
operator_policy :: binary() | none | undefined | '_', %% durable,
%% implicit
%% update
%% as above
gm_pids = [] :: [{pid(), pid()} | pid()] | none | '_', %% transient
decorators :: [atom()] | none | undefined | '_', %% transient,
%% recalculated
%% as above
state = live :: atom() | none | '_', %% durable (have we crashed?)
policy_version = 0 :: non_neg_integer() | '_',
slave_pids_pending_shutdown = [] :: [pid()] | '_',
vhost :: rabbit_types:vhost() | undefined | '_', %% secondary index
options = #{} :: map() | '_',
type = ?amqqueue_v1_type :: module() | '_',
type_state = #{} :: map() | '_'
}).
类型声明:
-type amqqueue() :: amqqueue_v1:amqqueue_v1() | amqqueue_v2().
-type amqqueue_v2() :: #amqqueue{
name :: rabbit_amqqueue:name(), %% 队列名称
durable :: boolean(), %% 是否为持久化队列
auto_delete :: boolean(), %% 是否自动删除
exclusive_owner :: pid() | none,
arguments :: rabbit_framing:amqp_table(), %% 属性参数
pid :: pid() | ra_server_id() | none,
slave_pids :: [pid()] | none,
sync_slave_pids :: [pid()] | none,
recoverable_slaves :: [atom()] | none,
policy :: binary() | none | undefined,
operator_policy :: binary() | none | undefined,
gm_pids :: [pid()] | none,
decorators :: [atom()] | none | undefined,
state :: atom() | none,
policy_version :: non_neg_integer(), %% 策略版本
slave_pids_pending_shutdown :: [pid()],
vhost :: rabbit_types:vhost() | undefined, %% 所在的虚
拟主机
options :: map(),
type :: atom(), %% 什么类型:disk还是ram的
type_state :: #{}
}.
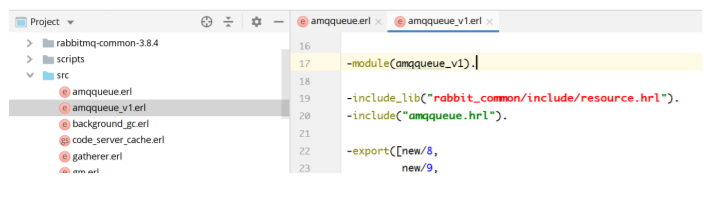
消息的确认:
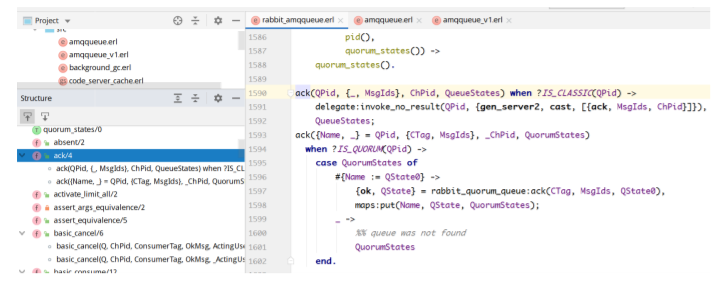
消息队列消费函数的声明:
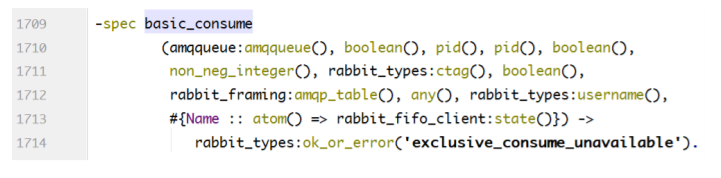
消费消息实现1:
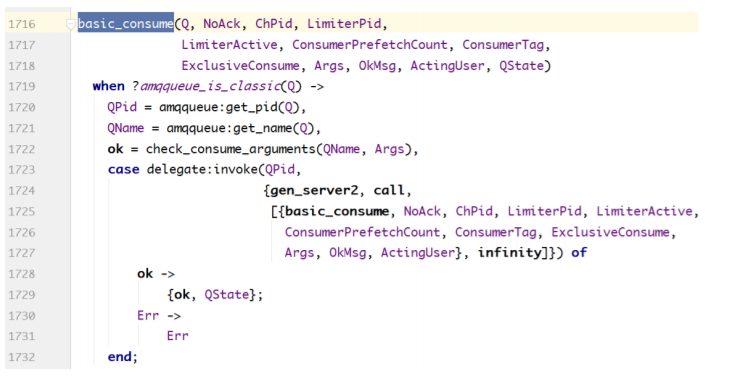
消费消息实现2:
basic_consume(Q, _NoAck, _ChPid,
_LimiterPid, true, _ConsumerPrefetchCount, _ConsumerTag,
_ExclusiveConsume, _Args, _OkMsg, _ActingUser, _QStates)
when ?amqqueue_is_quorum(Q) ->
{error, global_qos_not_supported_for_queue_type};
basic_consume(Q,
NoAck, ChPid, _LimiterPid, _LimiterActive, ConsumerPrefetchCount,
ConsumerTag, ExclusiveConsume, Args, OkMsg,
ActingUser, QStates)
when ?amqqueue_is_quorum(Q) ->
{Name, _} = Id = amqqueue:get_pid(Q),
QName = amqqueue:get_name(Q),
ok = check_consume_arguments(QName, Args),
QState0 = get_quorum_state(Id, QName, QStates),
case rabbit_quorum_queue:basic_consume(Q, NoAck, ChPid,
ConsumerPrefetchCount,
ConsumerTag,
ExclusiveConsume, Args,
ActingUser,
OkMsg, QState0) of
{ok, QState} ->
{ok, maps:put(Name, QState, QStates)};
{error, Reason} ->
rabbit_misc:protocol_error(internal_error,
"Cannot consume a message from quorum
queue '~s': ~w",
[rabbit_misc:rs(QName), Reason])
end.
消费消息实现3:
basic_consume(Q, _NoAck, _ChPid,
_LimiterPid, true, _ConsumerPrefetchCount, _ConsumerTag,
_ExclusiveConsume, _Args, _OkMsg, _ActingUser, _QStates)
when ?amqqueue_is_quorum(Q) ->
{error, global_qos_not_supported_for_queue_type};
主动拉消息函数声明:
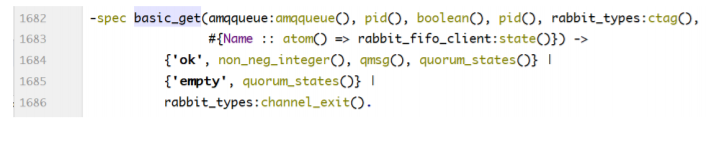
声明实现1:
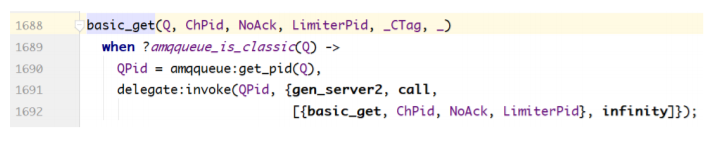
声明实现2:
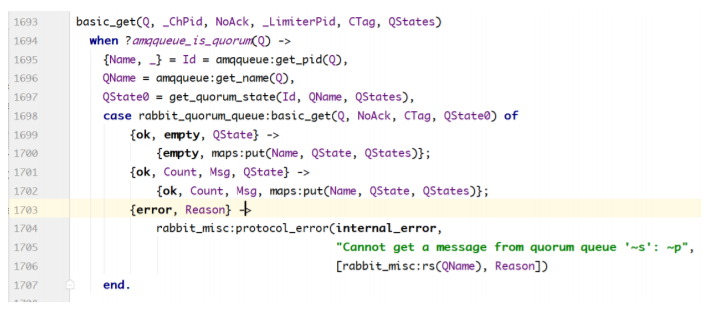
消息的确认:
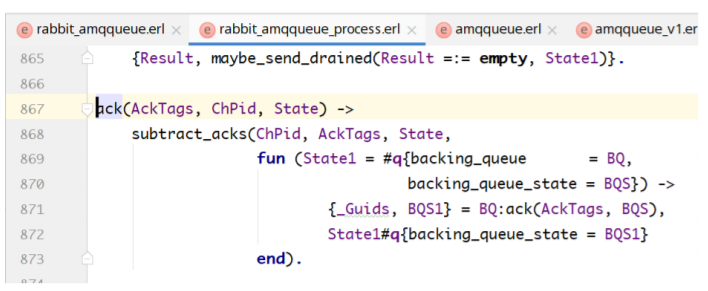
消息的重新入列:
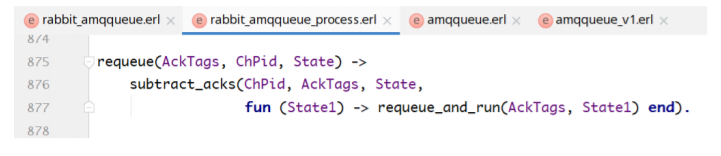
消息失效时间的计算:
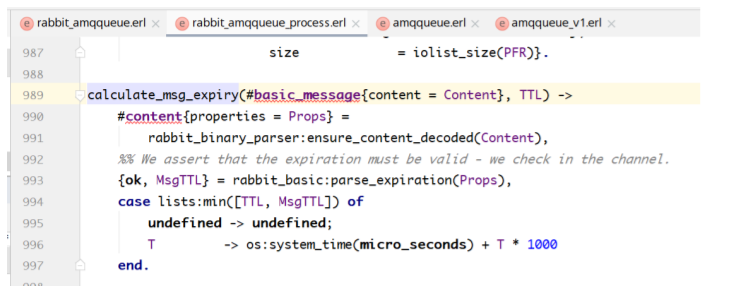
消息的发送确认:
confirm_messages(MsgIds, MTC) ->
{CMs, MTC1} =
lists:foldl(
fun(MsgId, {CMs, MTC0}) ->
case maps:get(MsgId, MTC0, none) of
none ->
{CMs, MTC0};
{SenderPid, MsgSeqNo} ->
{maps:update_with(SenderPid,
fun(MsgSeqNos) ->
[MsgSeqNo | MsgSeqNos]
end,
[MsgSeqNo],
CMs),
maps:remove(MsgId, MTC0)}
end
end, {#{}, MTC}, MsgIds),
maps:fold(
fun(Pid, MsgSeqNos, _) ->
rabbit_misc:confirm_to_sender(Pid, MsgSeqNos)
end,
ok,
CMs),
MTC1.
死信:
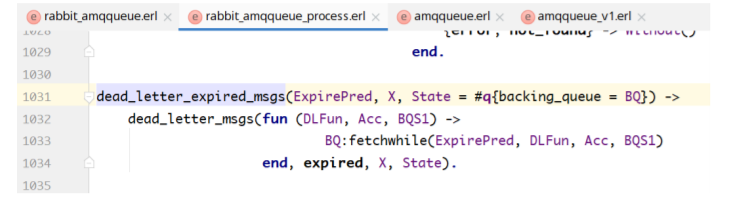
交换器
direct交换器
rabbit_exchange_type_direct.erl
看其中的路由方法:
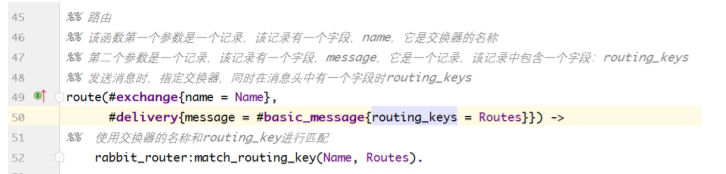
fanout交换器
rabbit_exchange_type_fanout.erl
看其中的路由方法:

head交换器
rabbit_exchange_type_headers.erl
看其中的路由方法:
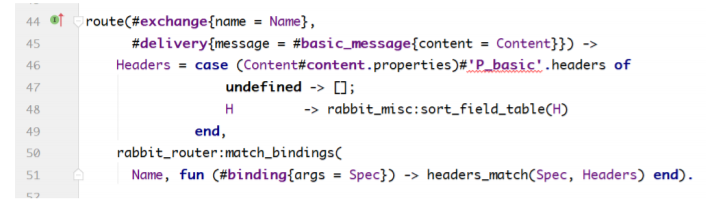
topic交换器
rabbit_exchange_type_topic.erl
看其中的路由方法:
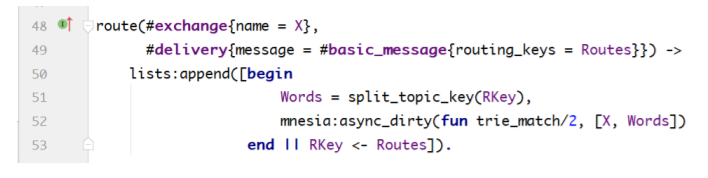
持久化
消息流转示意图:

rabbit_channel进程确定了消息将要投递的目标队列,rabbit_amqqueue_process是队列进程,每 个队列都有一个对应的进程,实际上rabbit_amqqueue_process进程只是提供了逻辑上对队列的相关 操作,他的真正操作是通过调用指定的backing_queue模块提供的相关接口实现的,默认情况该 backing_queue的实现模块为rabbit_variable_queue。 RabbitMQ队列中的消息随着系统的负载会不断 的变化,一个消息可能会处于以下4种状态:
%% Definitions:
%%
%% alpha: this is a message where both the message itself, and its
%% position within the queue are held in RAM(消息本身和消息位置索引都只在内存
中)
%%
%% beta: this is a message where the message itself is only held on
%% disk (if persisted to the message store) but its position
%% within the queue is held in RAM.(消息本身存储在磁盘中,但是消息的位置索引存在
内存中)
%%
%% gamma: this is a message where the message itself is only held on
%% disk, but its position is both in RAM and on disk.(消息本身存储在磁盘中,
但是消息的位置索引存在内存中和磁盘中)
%%
%% delta: this is a collection of messages, represented by a single
%% term, where the messages and their position are only held on
%% disk.(消息本身和消息的位置索引都值存储在磁盘中)
对于普通的没有设置优先级和镜像的队列来说,backing_queue的默认实现是 rabbit_variable_queue,其内部通过5个子队列Q1、Q2、Delta、Q3和Q4来实现这4个状态的转换, 其关系如下图所示:
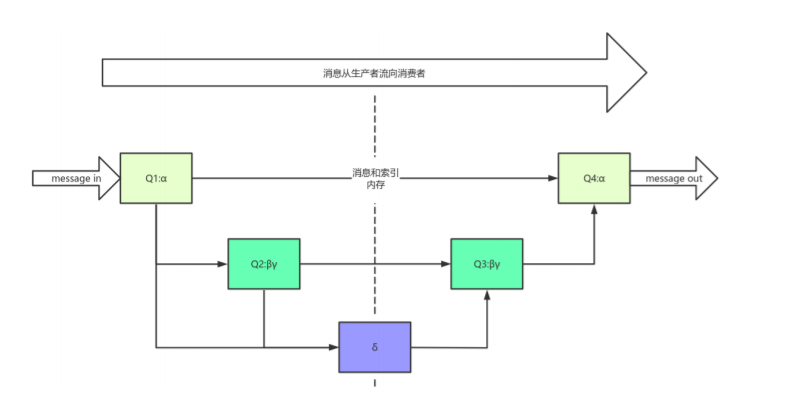
其中Q1、Q4只包含alpha状态的消息,Q2和Q3包含Beta和gamma状态的消息,Delta只包含 delta状态的消息。具体消息的状态转换后续会进行源码分析。
1 消息入队分析
rabbit_amqqueue_process对消息的主要处理逻辑位于deliver_or_enqueue函数,该方法将消息 直接传递给消费者,或者将消息存储到队列当中。
整体处理逻辑如下:
- 首先处理消息的mandory标志,和confirm属性。mandatory标志告诉服务器至少将该消息 route到一个队列中,否则将消息返还给生产者。confirm则是消息的发布确认。
- 然后判断队列中是否有消费者正在等待,如果有则直接调用backing_queue的接口给客户端发 送消息。
- 如果队列上没有消费者,根据当前相关设置判断消息是否需要丢弃,不需要丢弃的情况下调用 backing_queue的接口将消息入队。
deliver_or_enqueue函数代码:
deliver_or_enqueue(Delivery = #delivery{message = Message,
sender = SenderPid,
flow = Flow},
Delivered, State = #q{backing_queue = BQ,
backing_queue_state = BQS}) ->
%% 如果当前消息mandatory字段为true,则立刻通知该消息对应的rabbit_channel进程
send_mandatory(Delivery), %% must do this before confirms
%% 消息队列记录要confirm的消息,如果confirm为false,则不记录要confirm(如果消息需要进
行confirm,则将该消息的信息存入msg_id_to_channel字段中)
{Confirm, State1} = send_or_record_confirm(Delivery, State),
%% 得到消息特性特性数据结构
Props = message_properties(Message, Confirm, State1),
%% 让backing_queue去判断当前消息是否重复(rabbit_variable_queue没有实现,直接返回的
false)
{IsDuplicate, BQS1} = BQ:is_duplicate(Message, BQS),
State2 = State1#q{backing_queue_state = BQS1},
case IsDuplicate orelse attempt_delivery(Delivery, Props, Delivered,
State2) of
true ->
State2;
%% 已经将消息发送给消费者的情况
{delivered, State3} ->
State3;
%% The next one is an optimisation
%% 没有消费者来取消息的情况(discard:抛弃)
%% 当前消息没有发送到对应的消费者,同时当前队列中设置的消息过期时间为0,同时重新发送的
exchange交换机为undefined,则立刻将该消息丢弃掉
{undelivered, State3 = #q{ttl = 0, dlx = undefined,
backing_queue_state = BQS2,
msg_id_to_channel = MTC}} ->
%% 直接将消息丢弃掉,如果需要confirm的消息则立刻通知rabbit_channel进程进行
confirm操作
{BQS3, MTC1} = discard(Delivery, BQ, BQS2, MTC),
State3#q{backing_queue_state = BQS3, msg_id_to_channel = MTC1};
%% 没有消费者来取消息的情况
{undelivered, State3 = #q{backing_queue_state = BQS2}} ->
%% 将消息发布到backing_queue中
BQS3 = BQ:publish(Message, Props, Delivered, SenderPid, Flow, BQS2),
%% 判断当前队列中的消息数量超过上限或者消息的占的空间大小超过上限
{Dropped, State4 = #q{backing_queue_state = BQS4}} =
maybe_drop_head(State3#q{backing_queue_state = BQS3}),
%% 得到当前队列中的消息数量
QLen = BQ:len(BQS4),
%% optimisation: it would be perfectly safe to always
%% invoke drop_expired_msgs here, but that is expensive so
%% we only do that if a new message that might have an
%% expiry ends up at the head of the queue. If the head
%% remains unchanged, or if the newly published message
%% has no expiry and becomes the head of the queue then
%% the call is unnecessary.
case {Dropped, QLen =:= 1, Props#message_properties.expiry} of
%% 该情况是头部没有变化,同时消息队列消息树立不为一,则不管当前加入的消息是否
设置有超时时间,都不执行drop_expired_msgs函数
{false, false, _} -> State4;
%% 有丢弃消息,同时当前队列中只有当前这个新的消息,同时消息自己的特性过期时间
没有定义,则不检查消息过期
%% 此时消息的头部有变化,但是消息队列中只有一个消息,该消息还没有设置超时时
间,则不执行drop_expired_msgs函数
{true, true, undefined} -> State4;
%% 当向队列中插入消息后需要做检查消息过期,同时设置定时器的操作只有三种情况
%% 1.当消息头部根据队列上限有变化,同时消息插入后当前队列消息数量为一,且该
消息设置有过期时间,则需要做一次操作(该情况是消息头部有删除消息,都会进行一次消息过期检查)
%% 2.当消息头部根据队列上限有变化,同时消息插入后当前队列消息数量不为一,且
该消息设置有过期时间,则需要做一次操作(该情况是消息头部有删除消息,都会进行一次消息过期检查)
%% 3.当消息头部根据队列上限没有变化,同时消息插入后当前队列消息数量为一,不
管消息有没有过期时间,都要做一次操作(该情况下是当前队列进入第一条消息)
%% 最重要的是只要消息队列的头部消息有变化,则立刻执行drop_expired_msgs函
数,将队列头部超时的消息删除掉
{_, _, _} -> drop_expired_msgs(State4)
end
end.
如果调用到该方法的BQ:publish则说明当前队列没有消费者正在等待,消息将进入到队列。 backing_queue实现了消息的存储,他会尽力会durable=true的消息做持久化存储。初始默认情况下, 非持久化消息直接进入内存队列,此时效率最高,当内存占用逐渐达到一个阈值时,消息和消息索引逐 渐往磁盘中移动,随着消费者的不断消费,内存占用的减少,消息逐渐又从磁盘中被转到内存队列中。
消息在这些Queue中传递的"一般"过程q1->q2->delta->q3->q4,一般负载较轻的情况消息不需要 走完每个Queue,大部分都可以跳过。rabbit_variable_queue中消息的入队接口源码如下:
%% 消息的发布接口
publish(Msg = #basic_message { is_persistent = IsPersistent, id = MsgId },
MsgProps = #message_properties { needs_confirming = NeedsConfirming },
IsDelivered, _ChPid, _Flow,
State = #vqstate { q1 = Q1, q3 = Q3, q4 = Q4,
next_seq_id = SeqId,
in_counter = InCount,
durable = IsDurable,
unconfirmed = UC }) ->
%% 只有持久化队列和消息持久化才会对消息进行持久化
IsPersistent1 = IsDurable andalso IsPersistent,
%% 组装消息状态(该数据结构是实际存储在队列中的数据)
MsgStatus = msg_status(IsPersistent1, IsDelivered, SeqId, Msg, MsgProps),
%% 如果队列和消息都是持久化类型,则将消息内容和消息在队列中的索引写入磁盘
{MsgStatus1, State1} = maybe_write_to_disk(false, false, MsgStatus, State),
%% 将消息状态数据结构存入内存(如果Q3队列不为空,则将新消息存入Q1队列,如果为空则将新消息存
入Q4队列)
State2 = case ?QUEUE:is_empty(Q3) of
%% 如果Q3队列不为空,则将当前的消息写入Q1队列
false -> State1 #vqstate { q1 = ?QUEUE:in(m(MsgStatus1), Q1) };
%% 如果Q3队列为空,则将当前的消息写入Q4队列
true -> State1 #vqstate { q4 = ?QUEUE:in(m(MsgStatus1), Q4) }
end,
%% 进入队列中的消息数量加一
InCount1 = InCount + 1,
%% 如果消息需要确认,将该消息加入unconfirmed字段
UC1 = gb_sets_maybe_insert(NeedsConfirming, MsgId, UC),
%% 更新队列进程中的状态信息
State3 = stats({1, 0}, {none, MsgStatus1},
%% 更新下一个消息在消息中的位置
State2#vqstate{ next_seq_id = SeqId + 1,
in_counter = InCount1,
unconfirmed = UC1 }),
%% RabbitMQ系统中使用的内存过多,此操作是将内存中的队列数据写入到磁盘中
a(reduce_memory_use(maybe_update_rates(State3))).
消息入队时先判断Q3是否为空,如果Q3为空,则直接进入Q4,否则进入Q1,这里思考下为什么?
假如Q3为空,Delta一定为空,因为假如Delta不为空,那么Q3取出最后一个消息的时候Delta已经 把消息转移到Q3了,这样Q3就不是空了,前后矛盾因此Delta一定是空的。同理可以推测出Q2、Q1都 是空的,直接把消息放入Q4即可。
消息入队后,需要判断内存使用,调用reduce_memory_use函数:
每次入队消息后,判断RabbitMQ系统中使用的内存是否过多,此操作是尝试将内存中的队列数据 写入到磁盘中. 内存中的消息数量(RamMsgCount)及内存中的等待ack的消息数量(RamAckIndex) 的和大于允许的内存消息数量(TargetRamCount)时,多余数量的消息内容会被写到磁盘中.
2 消息出队源码分析
- 获取消息:
- 尝试从q4队列中获取一个消息,如果成功,则返回获取到的消息,如果失败,则尝试通过试 用fetch_from_q3/1从q3队列获取消息,成功则返回,如果为空则返回空;
- 注意fetch_from_q3从Q3获取消息,如果Q3为空,则说明整个队列都是空的,无消息,消费 者等待即可。
- 取出消息后:
- 如果Q4不为空,取出消息后直接返回;
- 如果Q4为空,Q3不为空,从Q3取出消息后,判断Q3是否为空,如果Q3为空,Delta不为 空,则将Delta中的消息转移到Q3中,下次直接从Q3消费;
- 如果Q3和Delta都是空的,则可以任务Delta和Q2的消息都是空的,此时将Q1的消息转移到 Q4,下次直接从Q4消费即可。
%% 从队列中获取消息
queue_out(State = #vqstate { q4 = Q4 }) ->
%% 首先尝试从Q4队列中取得元素(Q4队列中的消息类型为alpha)
case ?QUEUE:out(Q4) of
{empty, _Q4} ->
%% 如果Q4队列为空则从Q3队列中取得元素(如果Q3也为空,则直接返回空)
case fetch_from_q3(State) of
{empty, _State1} = Result -> Result;
{loaded, {MsgStatus, State1}} -> {{value, MsgStatus}, State1}
end;
{{value, MsgStatus}, Q4a} ->
{{value, MsgStatus}, State #vqstate { q4 = Q4a }}
end.
%% 从队列Q3中读取消息
fetch_from_q3(State = #vqstate { q1 = Q1,
q2 = Q2,
delta = #delta { count = DeltaCount },
q3 = Q3,
q4 = Q4 }) ->
%% 先从Q3队列中取元素(如果为空,则直接返回为空)
case ?QUEUE:out(Q3) of
{empty, _Q3} ->
{empty, State};
{{value, MsgStatus}, Q3a} ->
State1 = State #vqstate { q3 = Q3a },
State2 = case {?QUEUE:is_empty(Q3a), 0 == DeltaCount} of
{true, true} ->
%% 当这两个队列都为空时,可以确认q2也为空,也就是这时候,
q2,q3,delta,q4都为空,那么,q1队列的消息可以直接转移到q4,下次获取消息时就可以直接从q4获取
%% q3 is now empty, it wasn't before;
%% delta is still empty. So q2 must be
%% empty, and we know q4 is empty
%% otherwise we wouldn't be loading from
%% q3. As such, we can just set q4 to Q1.
%% 当Q3队列为空,且磁盘中的消息数量为空,则断言Q2队列为空
true = ?QUEUE:is_empty(Q2), %% ASSERTION
%% 当Q3队列为空,且磁盘中的消息数量为空,则断言Q4队列为空
true = ?QUEUE:is_empty(Q4), %% ASSERTION
%% 从Q3队列中取走消息后发现Q3队列为空,同时磁盘中没有消息,
则将Q1队列中的消息放入Q4队列
State1 #vqstate { q1 = ?QUEUE:new(), q4 = Q1 };
{true, false} ->
%% 从Q3队列中取走消息后发现Q3队列为空,q3空,delta非空,这
时候就需要从delta队列(内容与索引都在磁盘上,通过maybe_deltas_to_betas/1调用)读取消息,并
转移到q3队列
maybe_deltas_to_betas(State1);
{false, _} ->
%% q3非空,直接返回,下次获取消息还可以从q3获取
%% q3 still isn't empty, we've not
%% touched delta, so the invariants
%% between q1, q2, delta and q3 are
%% maintained
State1
end,
{loaded, {MsgStatus, State2}}
end.
转移Delta消息到Q3源码分析:
%% 从磁盘中读取队列数据到内存中来(从队列消息中最小索引ID读取出一个索引磁盘文件大小的消息索引信
息)
%% 从队列索引的磁盘文件将单个磁盘文件中的消息索引读取出来
%% 该操作是将单个队列索引磁盘文件中的deltas类型消息转换为beta类型的消息
maybe_deltas_to_betas(State = #vqstate {
q2 = Q2,
delta = Delta,
q3 = Q3,
index_state = IndexState,
ram_msg_count = RamMsgCount,
ram_bytes = RamBytes,
ram_pending_ack = RPA,
disk_pending_ack = DPA,
qi_pending_ack = QPA,
disk_read_count = DiskReadCount,
transient_threshold =
TransientThreshold }) ->
#delta { start_seq_id = DeltaSeqId,
count = DeltaCount,
end_seq_id = DeltaSeqIdEnd } = Delta,
%% 根据delta中的开始DeltaSeqId得到存在索引磁盘的最小的磁盘索引号
DeltaSeqId1 =
lists:min([rabbit_queue_index:next_segment_boundary(DeltaSeqId),
DeltaSeqIdEnd]),
%% 从队列索引中读取消息索引(从队列索引的磁盘文件将单个磁盘文件中的消息索引读取出来)
{List, IndexState1} = rabbit_queue_index:read(DeltaSeqId, DeltaSeqId1,
IndexState),
%% 过滤掉从rabbit_queue_index中读取过来的消息队列索引(如果该消息不是持久化的则需要删除
掉),最后得到当前内存中准备好的消息个数以及内存中的消息的总的大小
{Q3a, RamCountsInc, RamBytesInc, IndexState2} =
%% RabbitMQ系统关闭以前非持久化消息存储到磁盘中的索引信息再从磁盘读取出来的时候必须将
他们彻底从RabbitMQ系统中删除
betas_from_index_entries(List, TransientThreshold,
RPA, DPA, QPA, IndexState1),
%% 更新队列消息索引结构,内存中队列中的消息个数,队列内存中消息占的大小,以及从磁盘文件读取
的次数
State1 = State #vqstate { index_state = IndexState2,
ram_msg_count = RamMsgCount + RamCountsInc,
ram_bytes = RamBytes + RamBytesInc,
disk_read_count = DiskReadCount + RamCountsInc},
case ?QUEUE:len(Q3a) of
0 ->
%% we ignored every message in the segment due to it being
%% transient and below the threshold
%% 如果读取的当前消息队列索引磁盘文件中的操作项为空,则继续读下一个消息索引磁盘文
件中的操作项
maybe_deltas_to_betas(
State1 #vqstate {
delta = d(Delta #delta { start_seq_id =
DeltaSeqId1 })});
Q3aLen ->
%% 将从索引中读取出来的消息索引存储到Q3队列(将新从磁盘中读取的消息队列添加到老的
Q3队列的后面)
Q3b = ?QUEUE:join(Q3, Q3a),
case DeltaCount - Q3aLen of
0 ->
%% 如果读取出来的长度和队列索引的总长度相等,则delta信息被重置为消息个
数为0,同时q2中的消息转移到q3队列
%% delta is now empty, but it wasn't before, so
%% can now join q2 onto q3
State1 #vqstate { q2 = ?QUEUE:new(),
delta = ?BLANK_DELTA,
%% 如果磁盘中已经没有消息,则将Q2队列中的消息放入
Q3队列
q3 = ?QUEUE:join(Q3b, Q2) };
N when N > 0 ->
%% 得到最新的队列消息磁盘中的信息
Delta1 = d(#delta { start_seq_id = DeltaSeqId1,
count = N,
end_seq_id = DeltaSeqIdEnd }),
%% 更新最新的q3队列和磁盘信息结构
State1 #vqstate { delta = Delta1,
q3 = Q3b }
end
end.
3 总结
节点消息堆积较多时,这些堆积的消息很快就会进入很深的队列中去,这样会增加处理每个消息的 平均开销,整个系统的处理能力就会降低。因为要花更多的时间和资源处理堆积的消息,后流入的消息 又被挤压到很深的队列中了,系统负载越来越恶化。
因此RabbitMQ使用时一定要注意磁盘占用监控和流控监控,这些在控制台上都可以看到,一般来 说如果消息堆积过多建议增加消费者或者增强每个消费者的消费能力(比如调高prefetch_count消费者 一次收到的消息可以提高单个消费者消费能力)。
4 启动过程
看启动过程源码:
首先我们从一个脚本开始:启动RabbitMQ需要使用脚本:rabbitmq-server
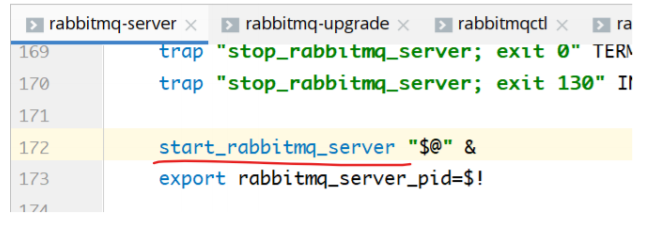
在172行调用了start_rabbitmq_server函数
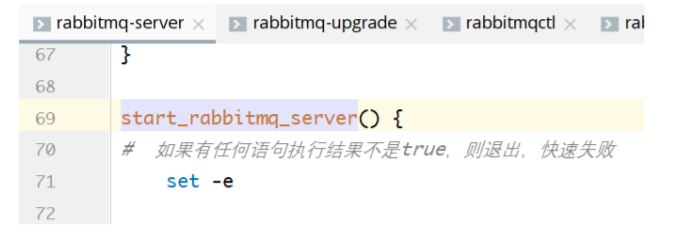
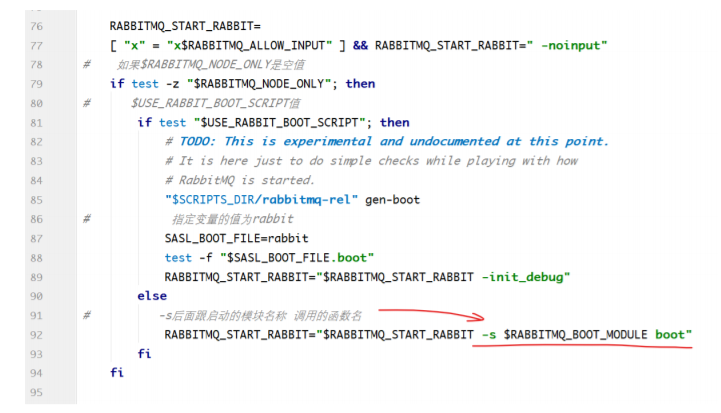
该函数在92行设置了RABBITMQ_START_RABBIT变量的值。
为什么是92行?因为在81行判断了环境变量USE_RABBIT_BOOT_SCRIPT的值,false。因为没有 USE_RABBIT_BOOT_SCRIPT环境变量。
这里在执行rabbitmq-server脚本的时候,在该脚本执行了一遍rabbitmq-env脚本,在rabbitmqenv中执行了一遍rabbitmq-defaults脚本。看完后发现没有USE_RABBIT_BOOT_SCRIPT。
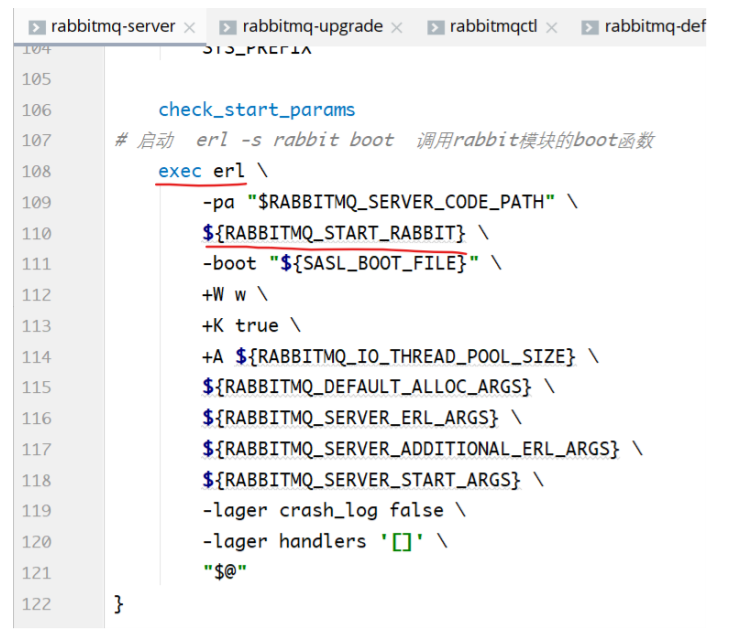
在第110行使用了RABBITMQ_START_RABBIT的扩展,也就是 -s rabbit boot ,它表示erlang要 调用rabbit模块的boot函数。
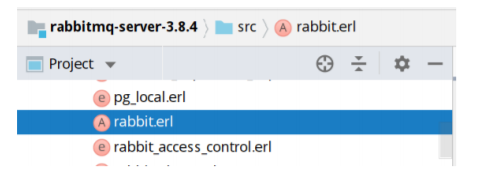
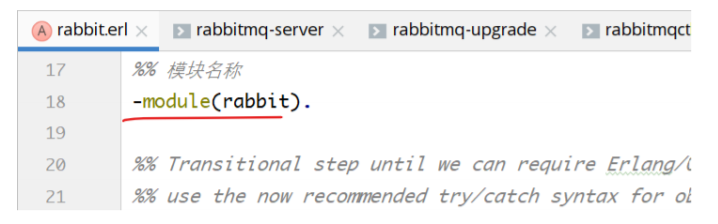
模块中的boot函数:
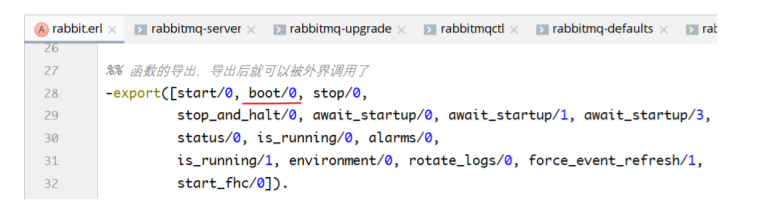
在模块中导出了boot/0函数。
boot/0函数的具体实现:
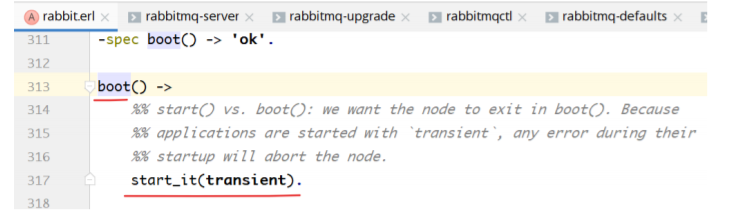
调用了start_it(transient)函数,参数的值就是transient。
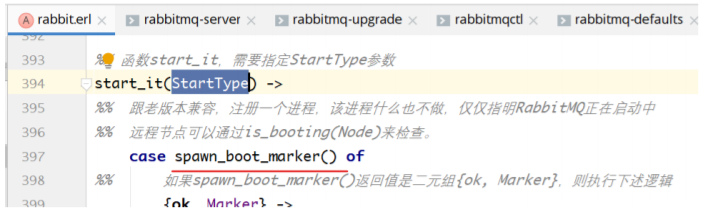
start_it函数首先调用了spawn_boot_marker()函数,然后对其结果做分支匹配。
spawn_boot_marker()函数:
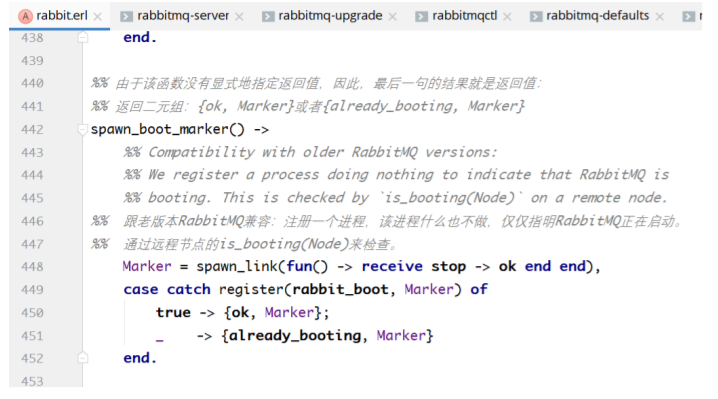
该函数什么也不做,仅仅是注册了一个进程,标志着RabbitMQ正在启动中。。。远程RabbitMQ 节点可以访问到这个状态。
该函数中调用了register函数,注册进程。如果注册成功了,则开始启动RabbitMQ,如果注册失 败,则表示RabbitMQ已经在启动中了。
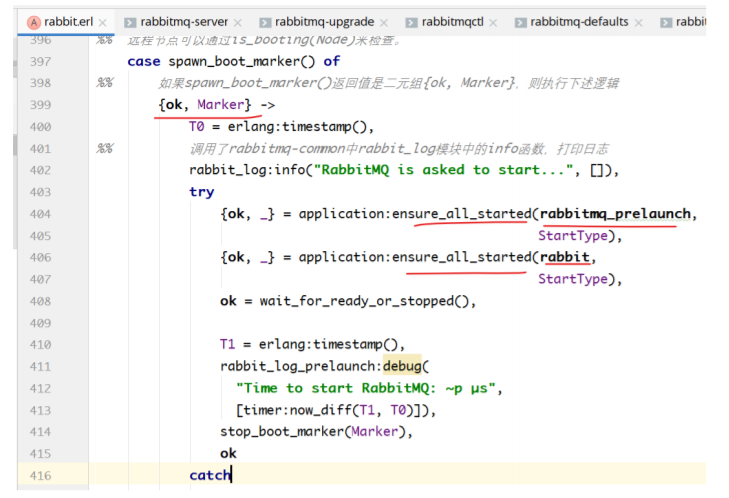
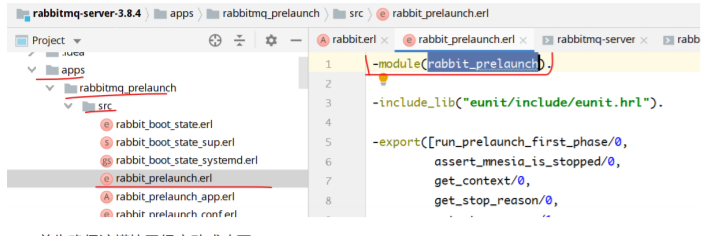
首先确保该模块已经启动成功了。
Erlang内核的application.erl:
ensure_all_started函数如下:
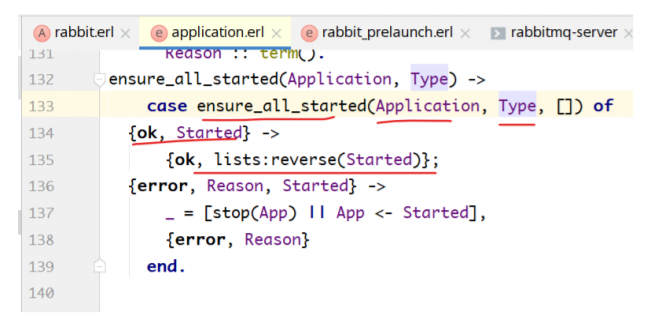
函数中调用了ensure_all_started函数:
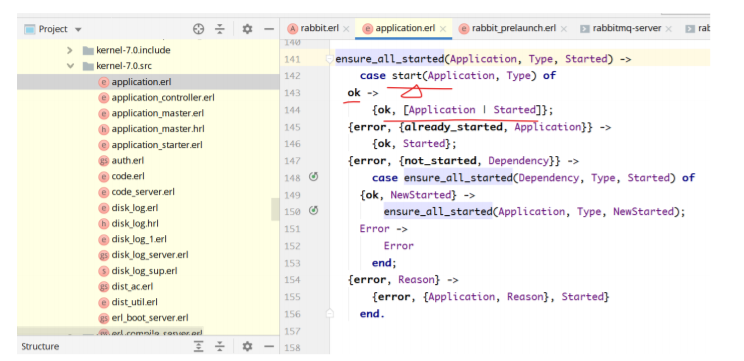
首先start该应用:rabbitmq_prelaunch
启动成功了,就返回{ok, [Application|Started]}
rabbit应用的ensure_all_started也是这个流程。
如果一切正常,rabbit和rabbitmq_prelaunch就都启动成功了。
启动的时候要回调rabbit的方法:start
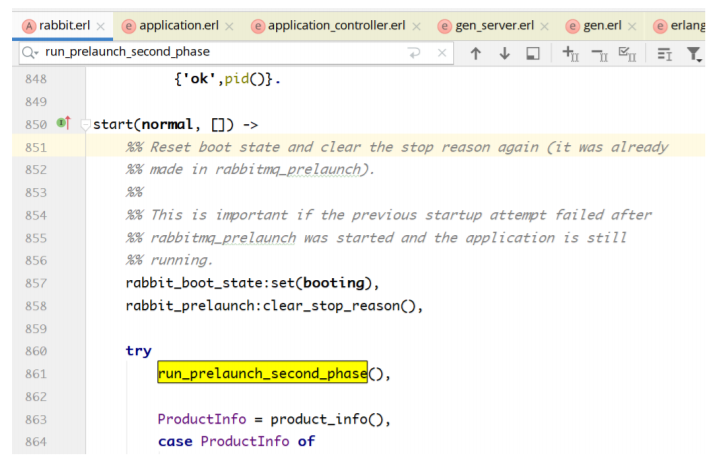
该方法中:
调用了run_prelaunch_second_phase()函数:
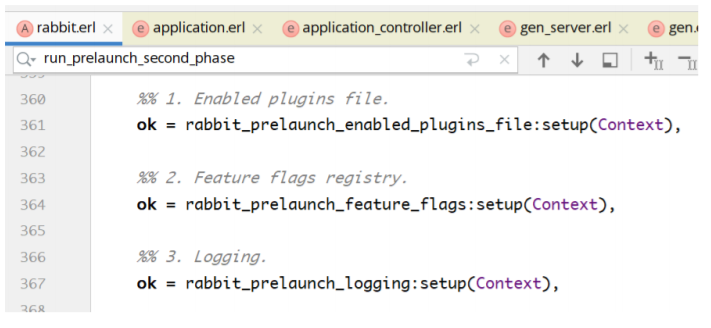
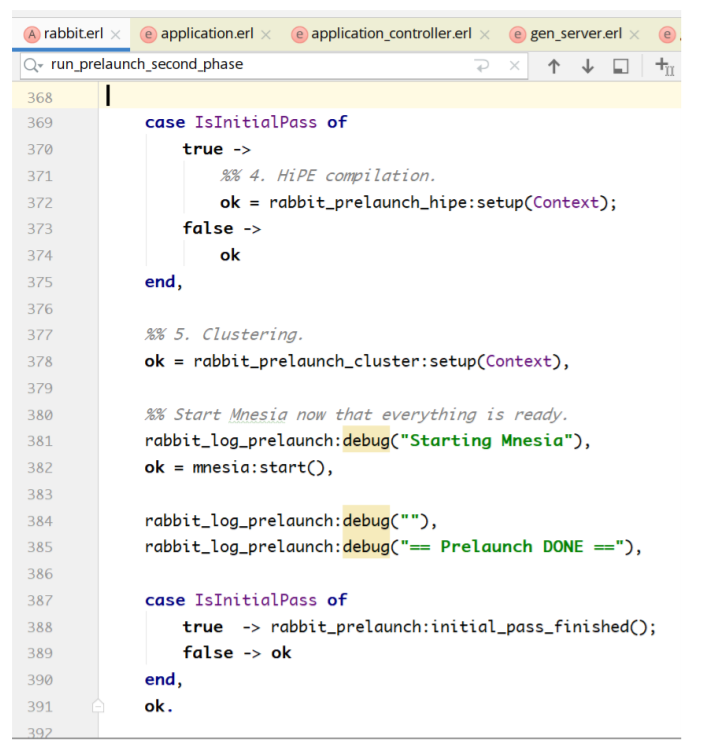
5 消息的发送
使用channel.basicPublish()方法发送消息:
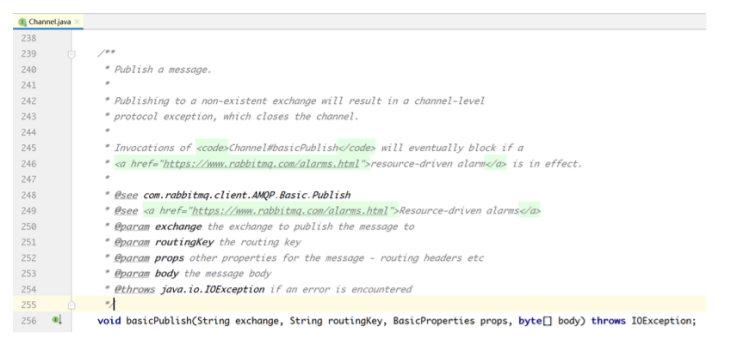
该抽象方法有如下实现:
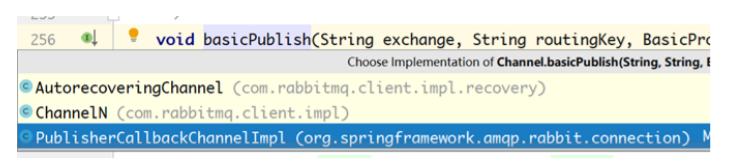
究竟是AutorecoveringChannel还是ChannelN还是PublisherCallbackChannelImpl,要看设置。
我们经常用的是ChannelN:
比如发送消息:
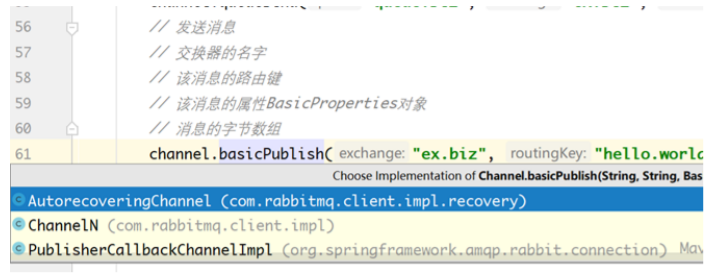
要看channel的来源:
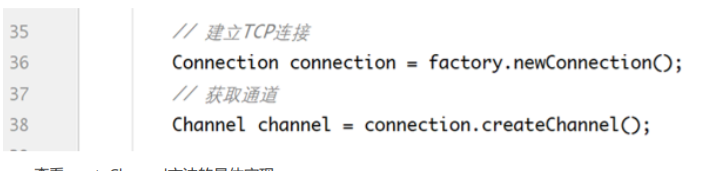
查看createChannel方法的具体实现:

究竟是AMQConnection还是AutorecoveringConnection?
需要看

打开该方法的实现:
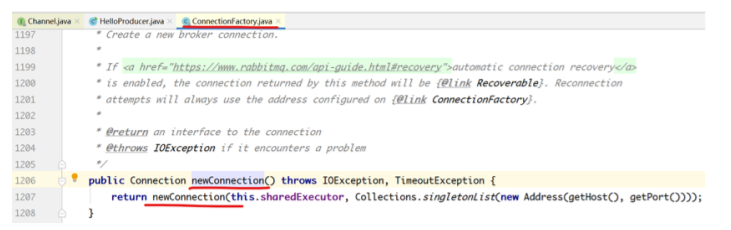
看newConnection方法的实现:
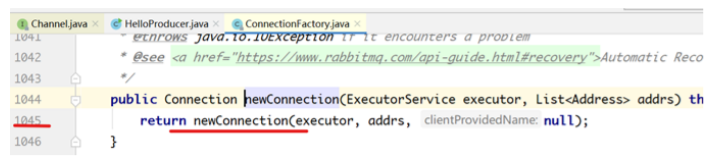
看newConnection方法的实现:
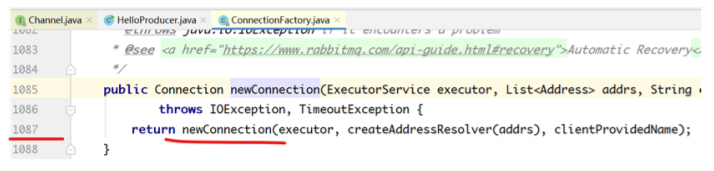
看newConnection方法的实现:
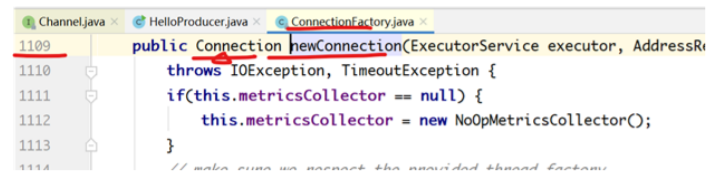
看哪里返回了Connection对象:
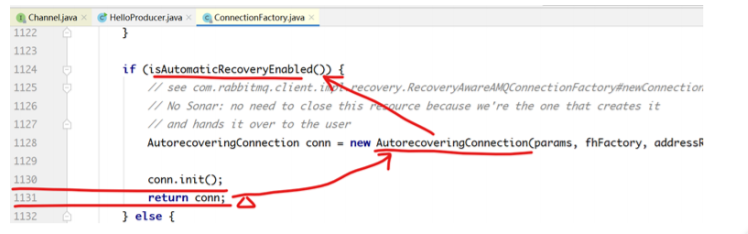
1131行返回Connection的AutorecoveringConnection对象。
前提是isAutomaticRecoveryEnabled()方法返回true。
该方法何时返回true?

如果在创建ConnectioFactory的时候设置了setAutomaticRecoveryEnabled为true,则1130行的 AutorecoveringConnection对象返回。
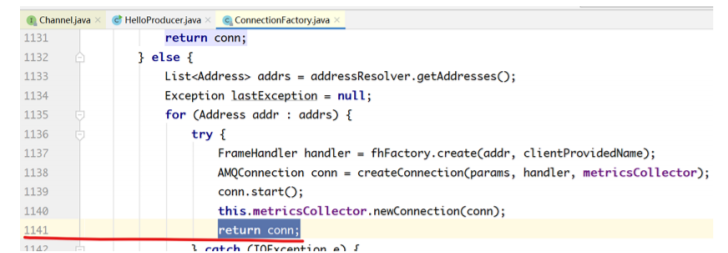
1141行返回AMQConnection对象。
在AMQConnection类中,查看createChannel()方法返回的Channel是哪个实现:
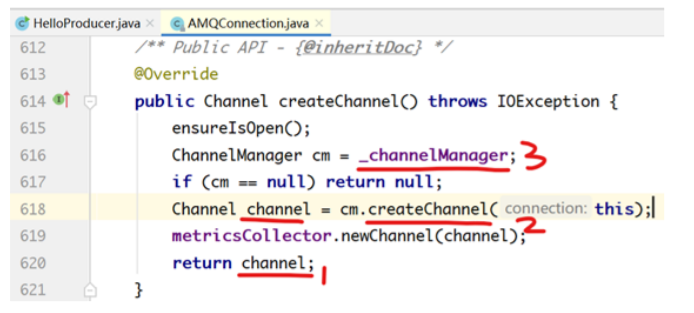
上述源码中,需要查看_channelManager的createChannel方法的返回值。
首先需要知道_channelManager是哪个类的对象:
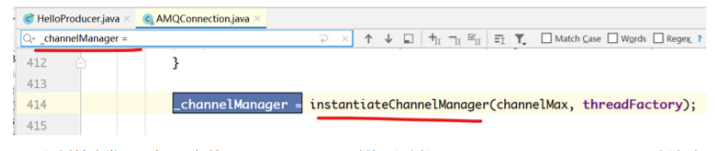
通过搜索发现只有414行给_channelManager赋值。通过调用instantiateChannelManager方法赋 值的。
看instantiateChannelManager的实现:
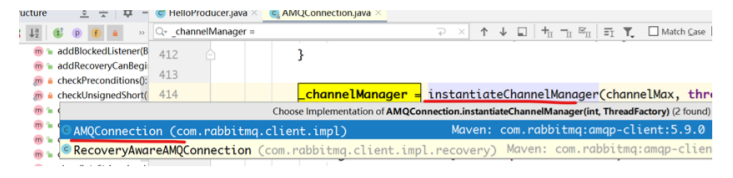
该方法有两个实现,我们查看AMQConnection中的实现:
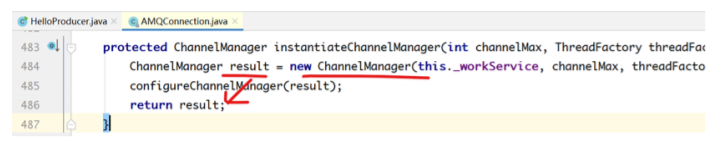
此处使用的是ChannelManager类。
回到前面:
看该类的createChannel方法返回的是哪个对象:
实现一:
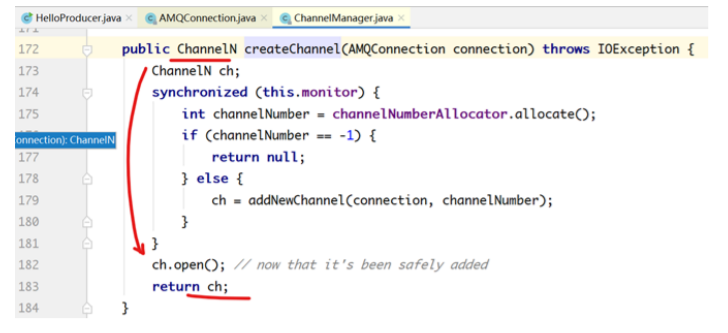
实现二:
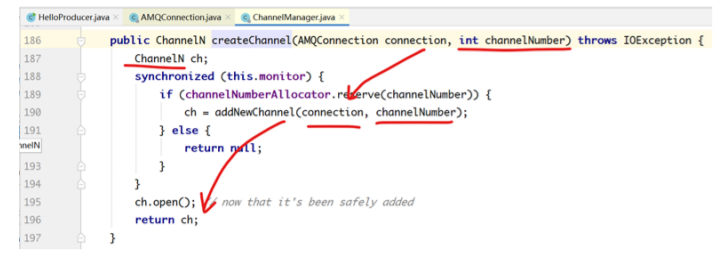
两个实现的区别在于有没有传递通道编号。
回到前面:
我们在发送消息的时候调用basicPublish方法,实际上就是ChannelN的方法:
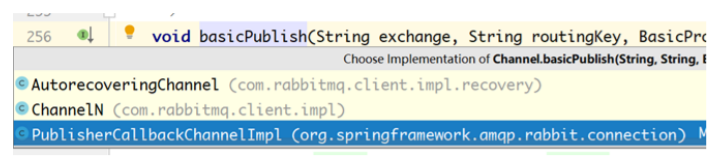
ChannelN中三个重载的basicPublish方法:
第一个方法:
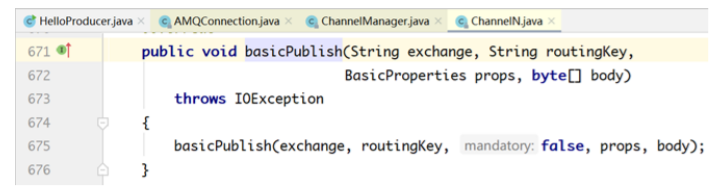
第二个方法:
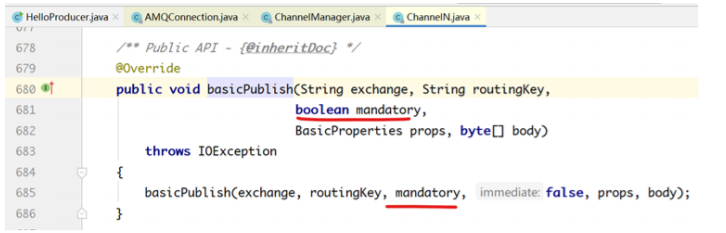
第三个方法:
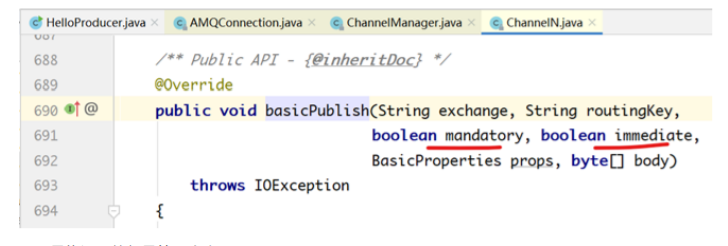
最终调用的都是第三个实现。
在第三个实现中,
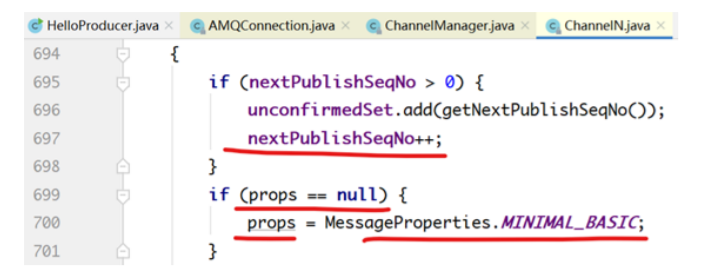
如果没有设置消息头,则设置最基本的消息头设置:
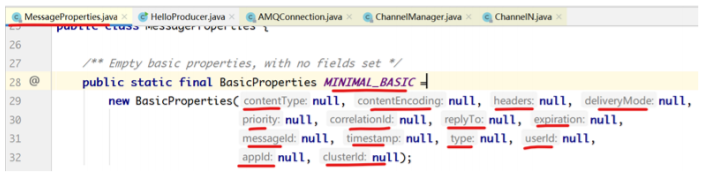
其中,
contentType表示内容类型,也就是MIME类型。
contentEncoding表示编码类型:如UTF-8
headers表示用户自定义的消息属性,键值对形式,Map
deliveryMode表示消息投递的模式,1表示瞬时消息,2表示持久化消息
priority表示消息的优先级,0~9,数字越大,优先级越高。
correlationId表示关联ID,一般用在RabbitMQ的请求/响应模式中,关联请求消息的ID
replyTo表示RabbitMQ的请求/响应模式中,响应消息要发送到的消息队列。
expiration表示消息的过期时间
messageId每个消息都有一个消息ID,该ID值要么手动设置,要么由系统自动生成,用于唯一标识 消息。
timestamp表示消息被发送的时间戳。这个时间戳并不是精确的消息被发送出的时间,而是在消息 放到发送队列到发送完成之间的任何时间。
type消息的类型,通常用语指定消息的序列化反序列化类型。
userId使用user-id属性来标识已登录的用户
appId在处理消息之前检查app-id允许应用程序丢弃那些来源不明或者不受支持的消息
clusterId:AMQP 0-9-1将cluster-id属性重新命名为reserved,并声明它必须为空,虽然 RabbitMQ目前没有根据规范要求它是空的,但是最好规避这个属性。
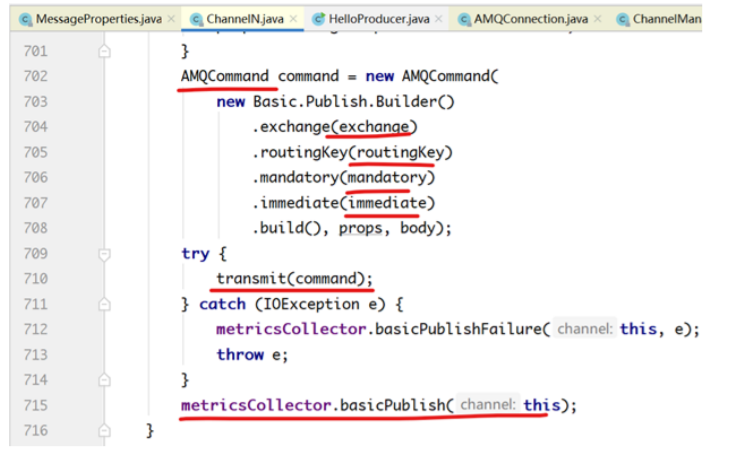
AMQCommand是AMQP规定的命令,用于跟RabbitMQ交互。命令中指定具体的操作,比如上文 中命令的属性是Basic.Publish,也就是AMQP的发布消息。
mandatory表示如果一个消息无法被交换器路由,则如果该值设置为0,则服务器悄无声息的丢 弃,否则使用AMQP的Return退还给发布者。
immediate如果该值设置为0,则当消息一到达交换器,就立即投递给消费者。如果消费者不在线 或不能立即投递给消费者,则服务器无法保证该消息被消费。如果设置为1,则如果消息不能被立即投 递给消费者,则使用AMQP的Return命令退还给发布者。
transmit用于执行该命令,发布消息。
最后一行用于发送统计消息。
transmit方法的实现:
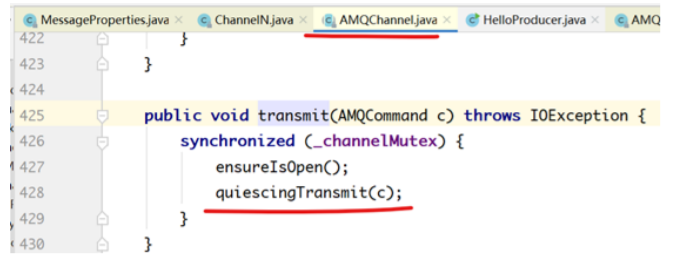
quiescingTransmit()用于执行AMQP命令:
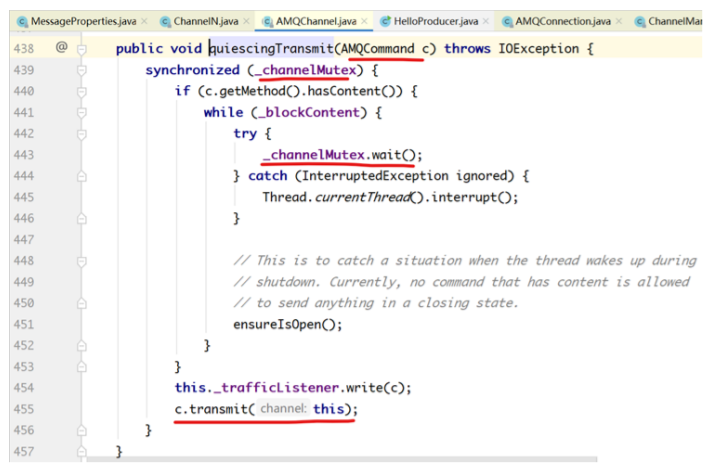
要在通道上执行命令,首先获取通道的共享锁,实际上就是一个Connection可以有多个通道来操 作,每个通道属于一个线程,连接是多线程共享的,因此需要获取该共享锁,以操作Connection。在 获取锁之后,如果此时发送线程需要阻塞,就让共享锁等待,直到被唤醒。
c.transmit(this)用于通过通道执行命令:
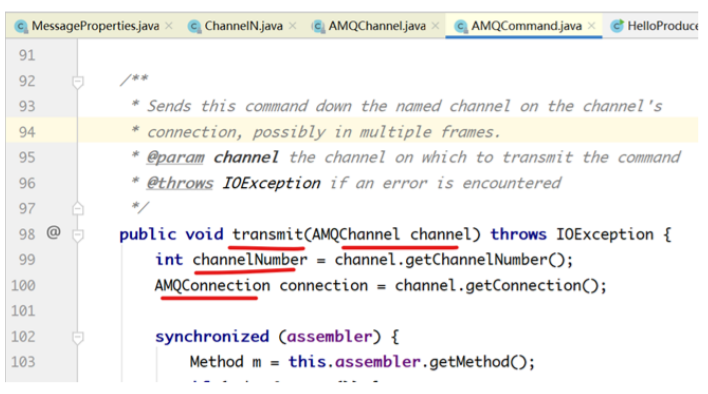
该方法首先获取通道号码,然后获取AMQConnection连接。
/**
* Sends this command down the named channel on the channel's
* connection, possibly in multiple frames.
* @param channel the channel on which to transmit the command
* @throws IOException if an error is encountered
*/
public void transmit(AMQChannel channel) throws IOException {
int channelNumber = channel.getChannelNumber();
AMQConnection connection = channel.getConnection();
synchronized (assembler) {
Method m = this.assembler.getMethod();
if (m.hasContent()) {
byte[] body = this.assembler.getContentBody();
Frame headerFrame =
this.assembler.getContentHeader().toFrame(channelNumber, body.length);
// 获取协议协商的帧大小
int frameMax = connection.getFrameMax();
boolean cappedFrameMax = frameMax > 0;
int bodyPayloadMax = cappedFrameMax ? frameMax - EMPTY_FRAME_SIZE :
body.length;
// 如果消息头帧大小大于协议协商的帧大小,则抛异常。
if (cappedFrameMax && headerFrame.size() > frameMax) {
String msg = String.format("Content headers exceeded max frame
size: %d > %d", headerFrame.size(), frameMax);
throw new IllegalArgumentException(msg);
}
// 发送要执行的AMQP方法
connection.writeFrame(m.toFrame(channelNumber));
// 发送消息头帧
connection.writeFrame(headerFrame);
// 封装消息帧,有可能有多个消息帧需要发送
for (int offset = 0; offset < body.length; offset += bodyPayloadMax)
{
int remaining = body.length - offset;
int fragmentLength = (remaining < bodyPayloadMax) ? remaining
: bodyPayloadMax;
Frame frame = Frame.fromBodyFragment(channelNumber, body,
offset, fragmentLength);
// 发送消息体帧,有可能多个
connection.writeFrame(frame);
}
} else {
// 如果要执行的AMQP方法没有数据,则只发送命令帧。
connection.writeFrame(m.toFrame(channelNumber));
}
}
connection.flush();
}
connection.writeFrame将消息帧发送到哪里了?
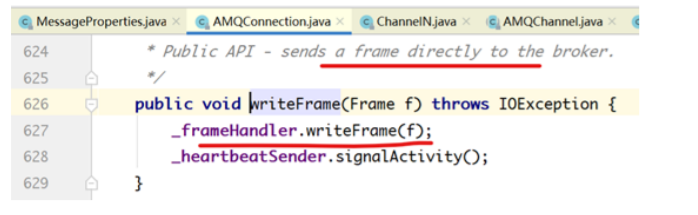
注释说直接将消息帧发送给broker,但实际上并非如此。
_frameHandler.writeFrame(f)用于写消息帧,写到哪里了?
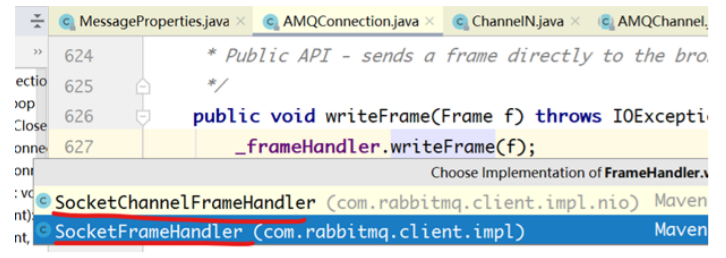
有两个实现,究竟是哪个?需要判断。且看_frameHandler的源码:
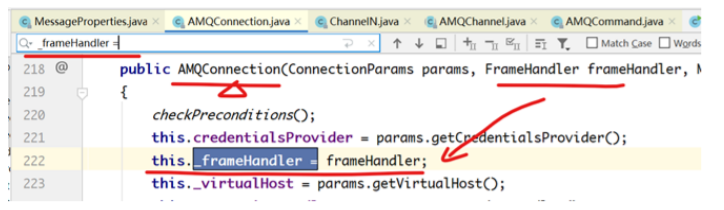
该属性只在构造器中初始化过,传过来的frameHandler是哪个?
要看在哪里创建AMQConnection对象的。
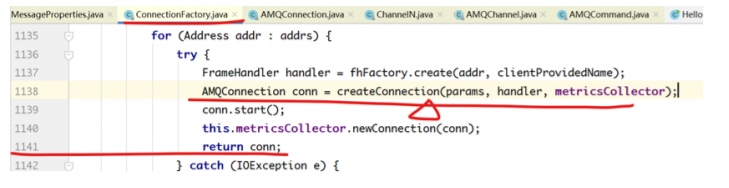
调用方法createConnection创建AMQConnection对象:

下图的FrameHandler对象是哪个?
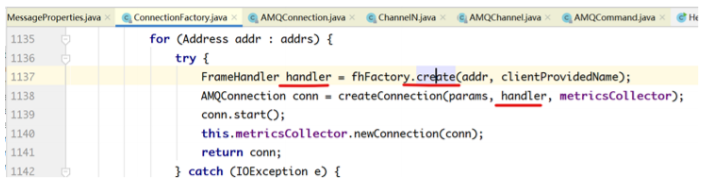
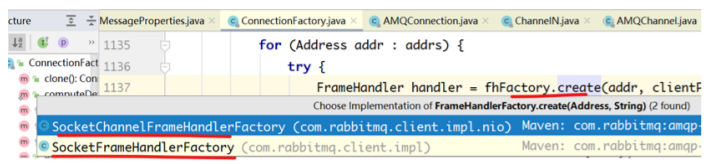
两个实现,该用哪个?
看fhFactory是哪个:
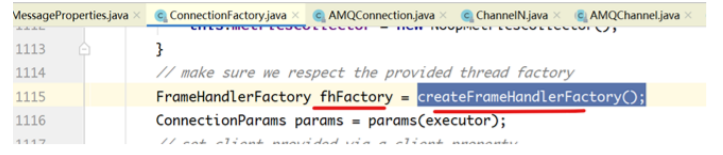
看createFrameHandlerFactory的实现:
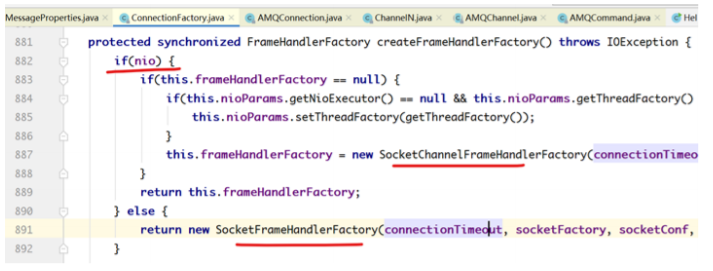
如果使用nio,则是SocketChannelFrameHandlerFactory,否则死 SocketFrameHandlerFactory。
看nio的值:
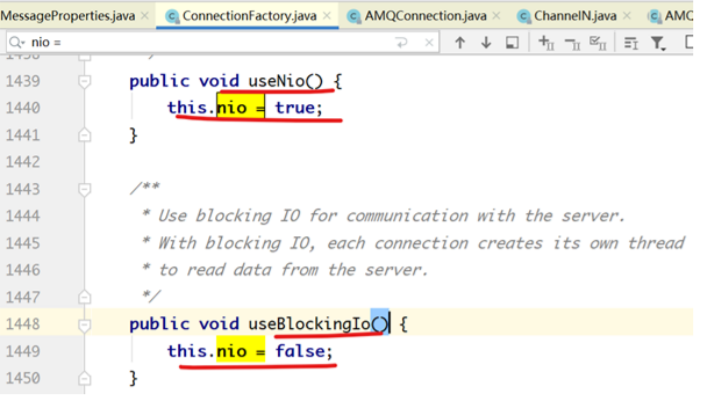
究竟调用了什么方法?
useNio还是useBlockingIo?
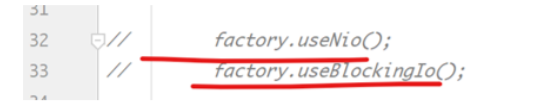
在我们的代码中可以手动调用。默认nio的值是:
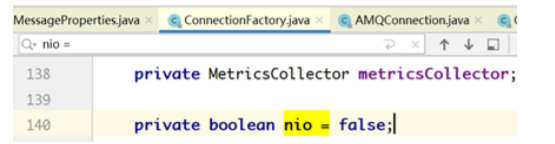
默认是false,非nio。
默认使用的就是SocketFrameHandlerFactory这个类。
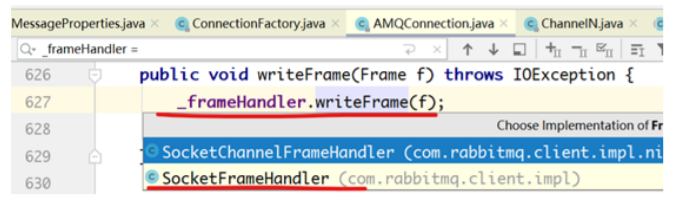
首先查看SocketFrameHandler的writeFrame实现:
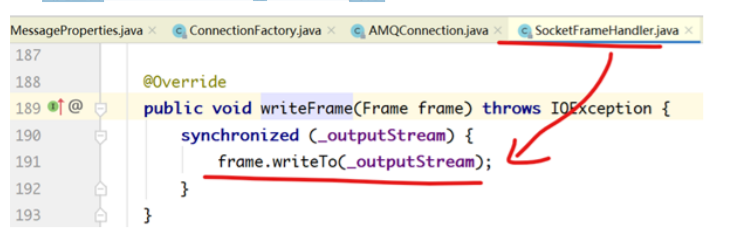
由于是阻塞IO,此处直接使用输出流输出:
输出流:_outputStream的赋值:
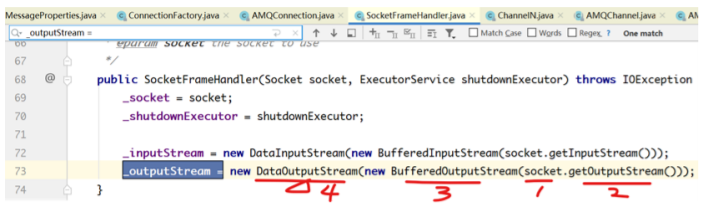
平淡无奇。
frame.writeTo(_outputStream)的实现:
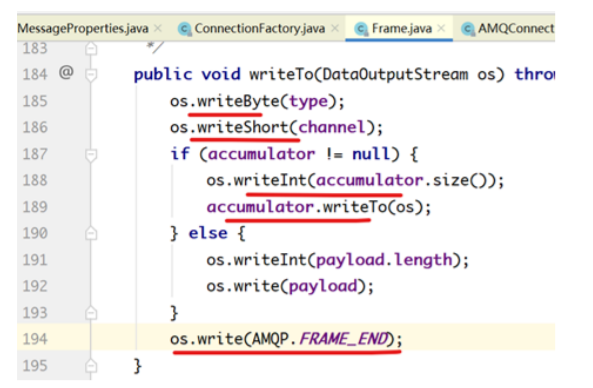
6 消息的消费
两种方式:推拉
拉消息:

推消息:

拉消息的代码实现

上图中,basicGet的具体实现是哪个?
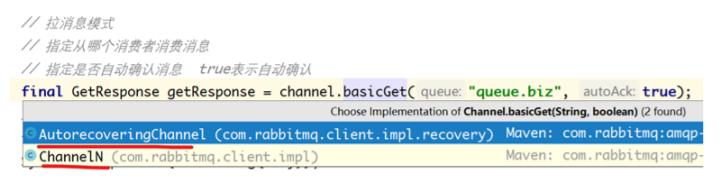
现在的Channel究竟是哪个类型?ChannelN还是AurecoveringChannel?

看该方法的返回值
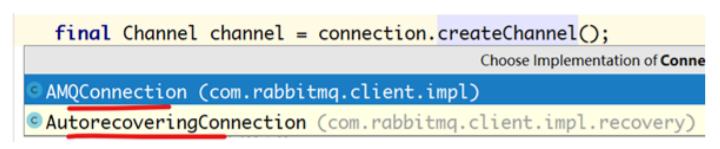
该方法在两个类中都存在,需要查看ConnectionFactory的方法返回的是哪个Connection:
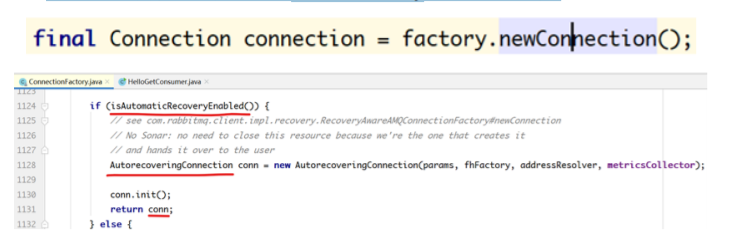
如果isAutomaticRecoveryEnabled()返回true,则返回的Connection是 AutorecoveringConnection的实例。
如果isAutomaticRecoveryEnabled()返回false, 则返回的是:
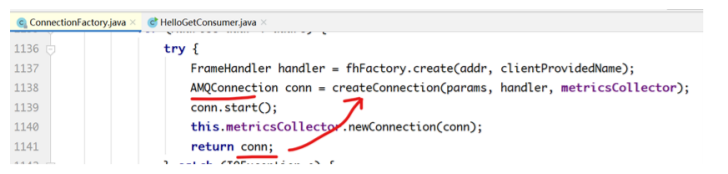
看createConnection方法的返回值是什么类型的:

就是AMQConnection类型的对象。
最简单的判断方式就是直接打印connection的class信息:
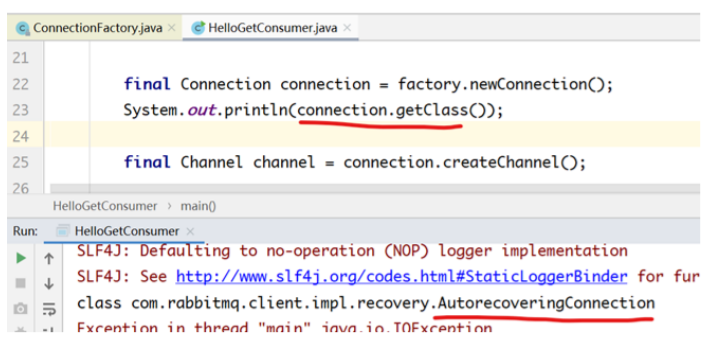
发现connection是AutoreceoveringConnection类型的对象。
isAutomaticRecoveryEnabled()返回的是true还是false?
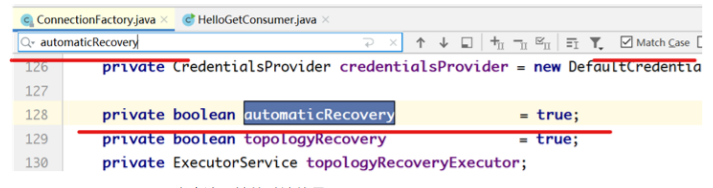
ConnectionFactory类中该属性的默认值是true。
还有两处涉及到变量automaticRecovery的:
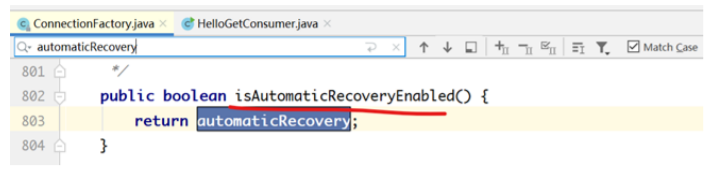
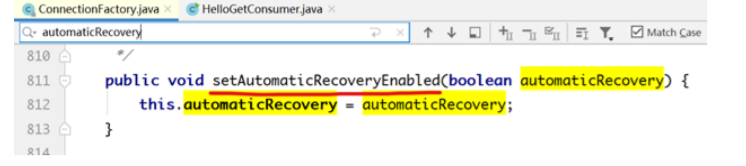
上图中的方法表示可以使用ConnectionFactory对象设置是否启用自动恢复特性。
默认Connection是AutorecoveringConnection类型的对象。
看下面的代码中channel的类型:

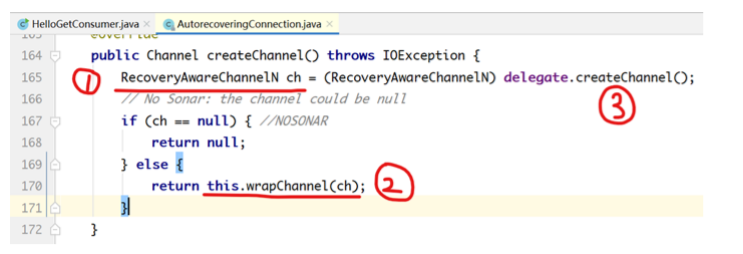
最终的返回值是wrapChannel方法调用的返回值:
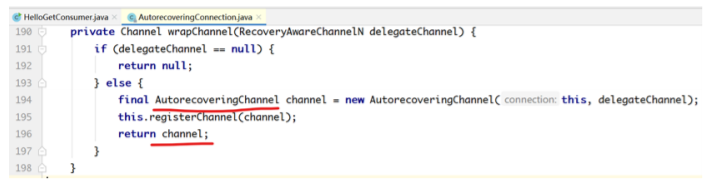
我们使用的channel的默认类型是AutorecoveringChannel。

看AutorecoveringChannel的basicGet实现:
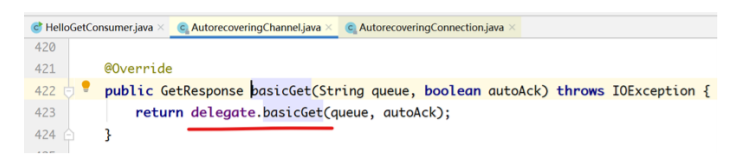
delegate是哪个?
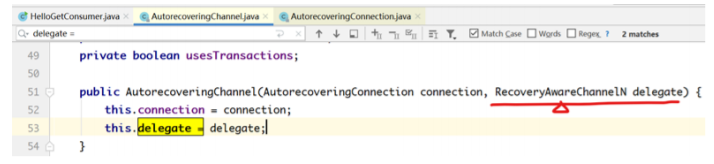
ChannelN.java中1149行是该方法的实现:
@Override
public GetResponse basicGet(String queue, boolean autoAck)
throws IOException
{
validateQueueNameLength(queue);
// 发送RPC请求,返回AMQCommand响应信息。
AMQCommand replyCommand = exnWrappingRpc(new Basic.Get.Builder()
.queue(queue)
.noAck(autoAck)
.build());
// 获取响应的方法
Method method = replyCommand.getMethod();
// 如果响应的方法是Basic.Ok类型的,则表示获取消息成功
if (method instanceof Basic.GetOk) {
// 向下转型
Basic.GetOk getOk = (Basic.GetOk)method;
// 使用Envelop封装响应的信息,包括消息ID,是否是重发的,交换器,路由键。
Envelope envelope = new Envelope(getOk.getDeliveryTag(),
getOk.getRedelivered(),
getOk.getExchange(),
getOk.getRoutingKey());
// 获取消息的属性
BasicProperties props =
(BasicProperties)replyCommand.getContentHeader();
// 获取消息体内容
byte[] body = replyCommand.getContentBody();
// 获取basic.getok.messagecount的值,此处是5
int messageCount = getOk.getMessageCount();
metricsCollector.consumedMessage(this, getOk.getDeliveryTag(), autoAck);
// 实例化GetResponse对象并赋值返回。
return new GetResponse(envelope, props, body, messageCount);
} else if (method instanceof Basic.GetEmpty) {
return null;
} else {
throw new UnexpectedMethodError(method);
}
}
如何获取消息的?发送RPC请求:
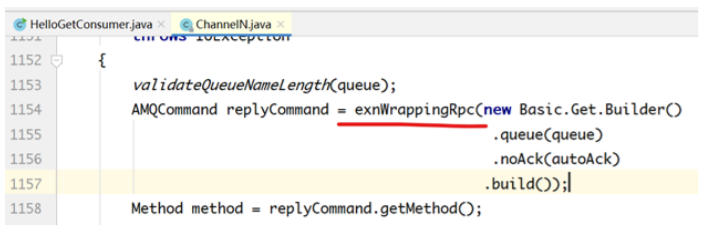
看该方法的实现:
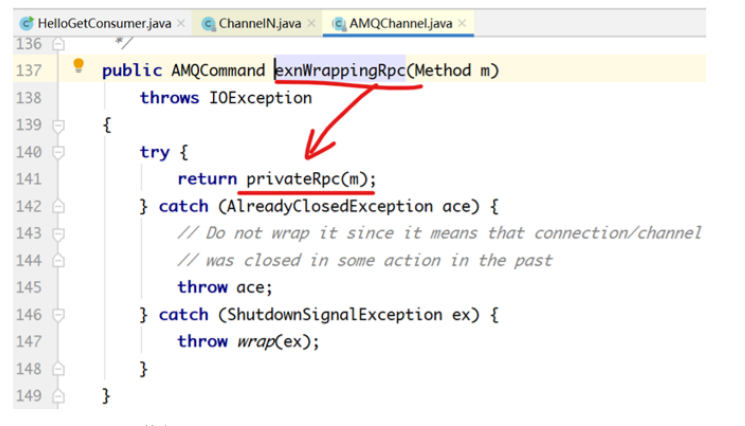
privateRpc(m)的实现:
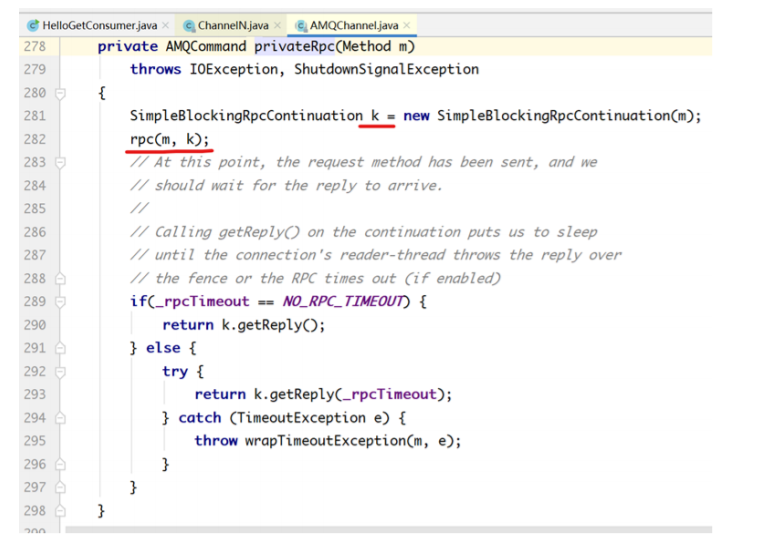
上述代码中,rpc(m, k)发送请求消息。
k.getReply()方法是一个阻塞的方法,等待broker返回响应。
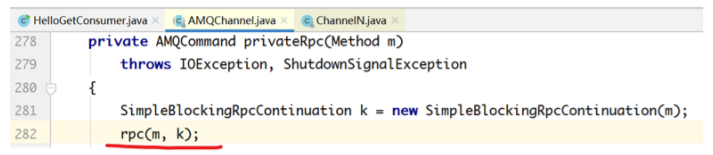
rpc方法的具体私实现:
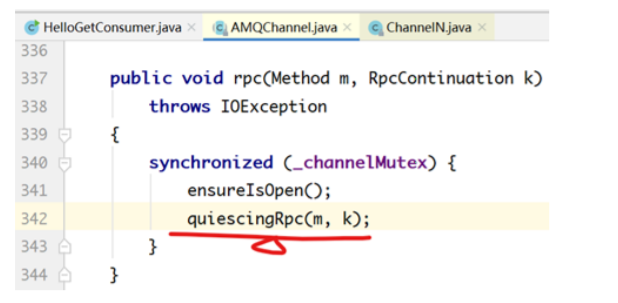
quiescingRpc(m, k)的具体实现:
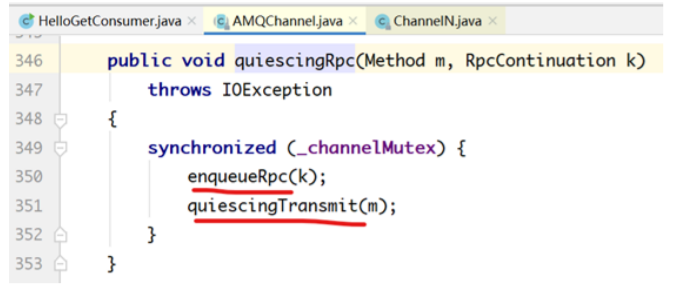
enqueueRpc(k)具体实现:
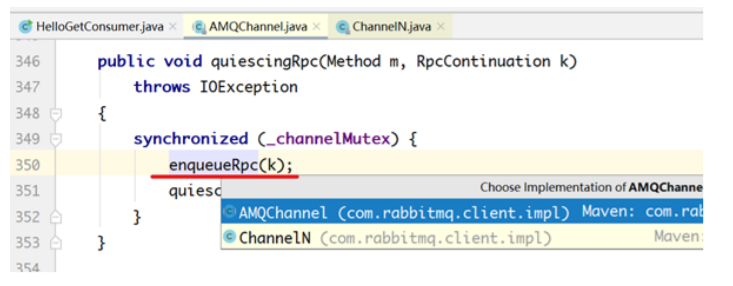
我们使用的channel的默认实现是:AutorecoveringChannel,该类中包含

RecoveryAwareChannelN是
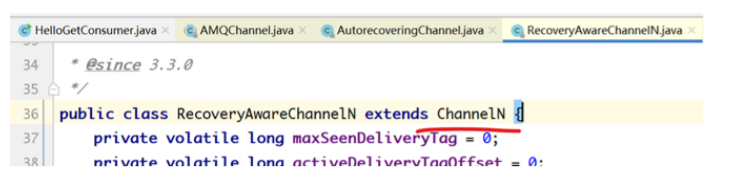
ChannelN的子类。
ChannelN又是AMQChannel的子类。
所以enqueueRpc方法应该看ChannelN的实现方式:
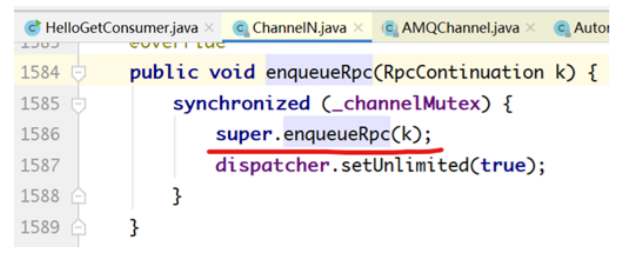
调用了父类的enqueueRpc方法是父类的:
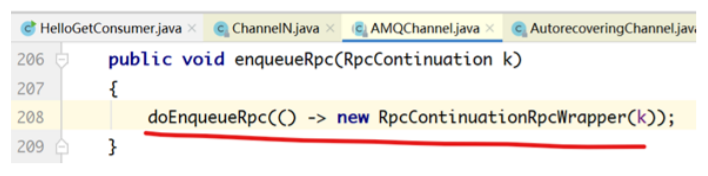
看doEnqueueRpc的具体实现:
推消息的代码实现

看basicConsume的具体实现:
ChannelN中1343行代码:
public String basicConsume(String queue, final boolean autoAck, String
consumerTag,
boolean noLocal, boolean exclusive, Map<String,
Object> arguments, final Consumer callback) throws IOException {
// 构建请求方法
final Method m = new Basic.Consume.Builder()
.queue(queue)
.consumerTag(consumerTag)
.noLocal(noLocal)
.noAck(autoAck)
.exclusive(exclusive)
.arguments(arguments)
.build();
BlockingRpcContinuation<String> k = new BlockingRpcContinuation<String>(m) {
@Override
public String transformReply(AMQCommand replyCommand) {
String actualConsumerTag = ((Basic.ConsumeOk)
replyCommand.getMethod()).getConsumerTag();
_consumers.put(actualConsumerTag, callback);
// need to register consumer in stats before it actually starts
consuming
metricsCollector.basicConsume(ChannelN.this, actualConsumerTag,
autoAck);
dispatcher.handleConsumeOk(callback, actualConsumerTag);
return actualConsumerTag;
}
};
rpc(m, k);
try {
if(_rpcTimeout == NO_RPC_TIMEOUT) {
return k.getReply();
} else {
try {
return k.getReply(_rpcTimeout);
} catch (TimeoutException e) {
throw wrapTimeoutException(m, e);
}
}
} catch(ShutdownSignalException ex) {
throw wrap(ex);
}
}